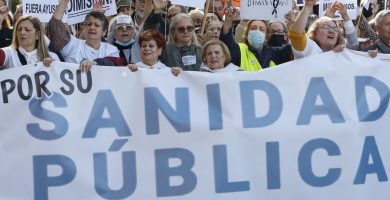
Lo que le duele al doctor: condiciones laborales de los médicos de Atención Primaria.
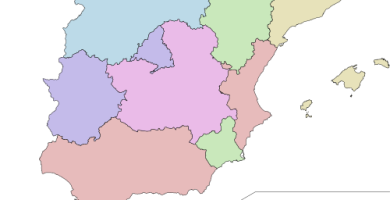
C.C.A.A. en las que mejor funciona la Sanidad Pública
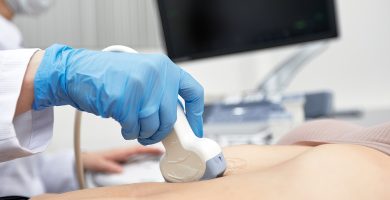
Los mejores ginecólogos de España
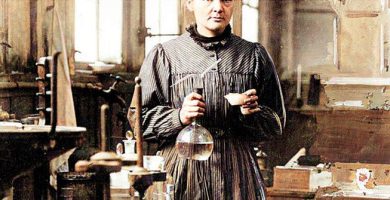
Mujeres influyentes en la historia de la medicina
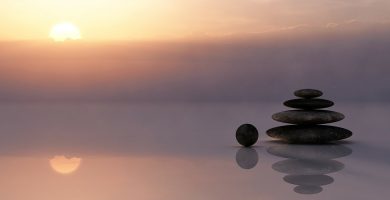
Consejos para el cuidado emocional de médicos y sanitarios
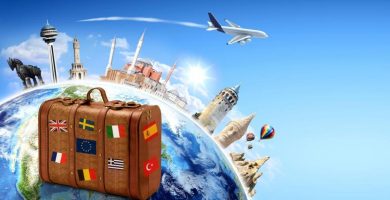
Los mejores paÃses para trabajar de enfermero
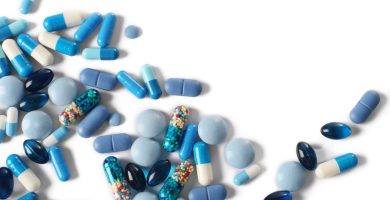